A COMPARATIVE ANALYSIS OF EXTREME GRADIENT BOOSTING AND SUPPORT VECTOR REGRESSION FOR MODELING BENCHMARK CRUDE OIL PRICES
Keywords:
Ensemble Algorithms, XGBoosting, ICE Brent, NYMEX WTI, ORB, OPEC, OECD Support Vector RegressionAbstract
Organization of Petroleum Exporting Countries (OPEC) and non-OPEC supply, oil balance, oil demand by Organization for Economic Cooperation and Development (OECD) and non-OECD members, money market managers' long positions, US consumer price index and spot prices of crude oils like New York Mercantile Exchange West Texas Intermediate (NYMEX WTI), Intercontinental Exchange (ICE) Brent, OPEC Reference Basket (ORB), and other crude oils are basic elements driving the patterns seen in the market pricing of crude oils. Data between 2008 and 2022 were obtained for this study from OPEC Monthly Oil Market Reports. This research evaluates the performance of two machine learning models, Support Vector Regression (SVR) and Extreme Gradient Boosting (XGBoost), in predicting crude oil prices for three major benchmarks: OPEC Reference Basket (ORB), NYMEX WTI, and ICE Brent. Using key performance metrics such as Mean Squared Error (MSE), Root Mean Squared Error (RMSE), and R-squared (R²), the study highlights the strengths and weaknesses of each model in both stable and volatile market conditions. SVR shows strong predictive accuracy, particularly for ICE Brent, but struggles with price volatility in the ORB and NYMEX WTI datasets. XGBoost is more robust in handling volatility and non-linear relationships. The findings have important economic implications for market participants, suggesting that while SVR is suited for stable pricing environments, XGBoost is better equipped to handle the unpredictability of more volatile markets.
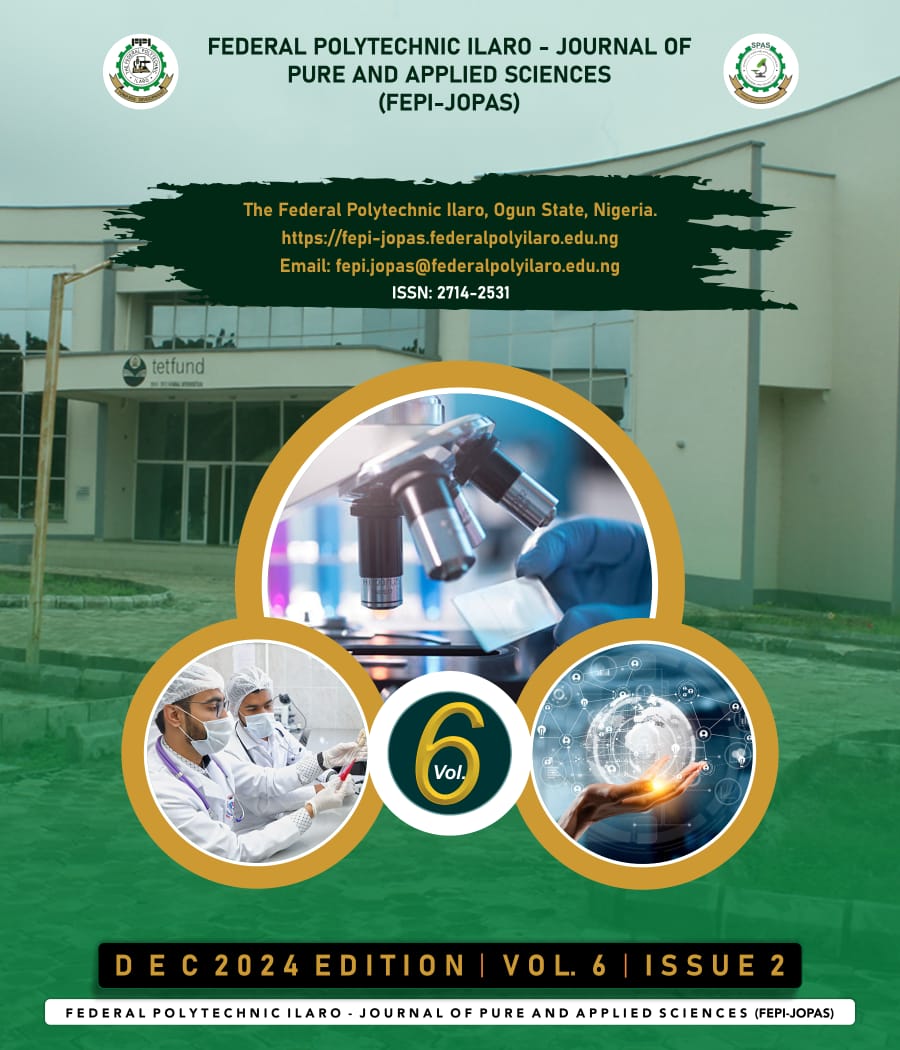